![]() |
Supercomputer; Wikimedia |
And then a physicist in the room spoke up. He didn't understand why people were so pessimistic about our ability to eventually understand the disease. Or any disease. He himself is certain that with the incredible computing power we've got now we'll be able to understand, and predict, them all. A few others in the room signed on to that, none of them physicists, but similarly optimistic.
A piece has just been published at Wired online (18 March 2013) that says much the same. Richard Barker: "Illness just became another big data problem." Barker describes the case of an infant born with a rare form of type 1 diabetes for whom the proper treatment is begun once the child is genotyped and his particular mutation identified.
So diabetes isn't just diabetes: it's a cluster of diseases with different causes and different remedies. This story is just a glimpse of a quiet medical revolution: from defining diseases by the symptoms they cause or the part of the body affected, to the underlying molecular mechanism.Further, if this child had been sequenced at birth, as our children, or grandchildren, or great-grandchildren will be, his illness would have been identified before he was even ill, and his treatment would have been started immediately. This day is coming.
An anthropological question: fad, fact, or cultural bias?
This all sounds very hopeful. But history shows how at any given era there is a working model of how to do things, and basically everyone except mavericks follow suit. We want to be in on things, to be doing what seems right, to have support from our fellows, and the comfort all of this brings. We often stick to this even if there is no real evidence that it is working or evidence that it may not be the best approach. The long-lasting nature of Galenic (four-humours) medicine is one example. Armies routinely train for the last war, and pay a price for it. Religions, though supposedly based on ultimate divinely given truths, form sects and alter their doctrine.
At the same time, it may be--especially in science--that the current way, though imperfect, is the result of centuries of improvement and is the best we can do at any given time. Certainly we'll not just quit because we know our methods have imperfections! So, it is fair to ask: is illness now just a data crunching problem?
Well, we can pretty much eliminate infectious diseases right off the bat. While there may be identifiable genetic susceptibility that explain a small minority of resistance to some infectious diseases, this is by far overwhelmed by other factors that predispose people to infection, like poverty and bad luck. That makes a lot of illness around the world not reducible to a data crunching, at the individual level. There's certainly a lot of population-based data crunching that can model risk and explain it in populations, but no amount of sequencing of infants at birth will identify those who will be at highest risk come the next epidemic.
Then there are complex diseases, like heart disease, or type 2 diabetes or asthma or schizophrenia or autism or hypertension or stroke or most cancers. Sequencing can't now, and although it's not fashionable to say so, many believe will never be able to predict accurately who's at risk of most of these diseases, for reasons that we write about here all the time. They're complex, they're polygenic, everyone has a different and unique genome, and a different pathway to disease, environmental factors are causal, and inherently unpredictable. And so forth.
So now we've pretty much eliminated the big causes of death around the world from the illness as big data problem model. What's left?
Mendelian diseases, like the form of diabetes Barker described, or the thousands of other primarily very rare and usually pediatric diseases that really are genetic, many of which are now, and will eventually be, identifiable and usually (but not always) predictable with genetic data. But, many such diseases and disorders are themselves very complex -- cystic fibrosis is a well-studied and well-characterized example, with over 1000 different implicated alleles, all in the same gene, identified to date.
Studies of unexplained genetic disorders, that is where there is familial risk and some cases have an identified gene, have about a 25% success rate for identifying a causal mutation. Granted, by now it's probably the toughest, rarest disorders that are left to explain -- and/or those without effective enough advocacy groups to have lobbied for funding; but some of these will be explained, while others will not, because there can be numerous pathways to the same phenotype, and what's causal for one individual won't explain it in another. This is something we know very well already.
![]() |
Late spring wildflowers; Wikimedia |
She tells the story of how it had been believed that the Neanderthal buried their dead with flowers, or may even have used flowers medicinally, based on pollen finds in caves in which skeletons were found. It was a beautiful idea, she writes, except that it was probably wrong. The pollen was more likely blown in by the wind, or carried in on a rodent's fur.
I confess to finding this story pleasing, not disappointing. It exposes the depths of our fantasies about people and plants, showing how pattern-driven we are, and how addicted to stories. At the same time, it reveals the baffling complexity of the natural world, its resistance to understanding. No matter what meanings we ascribe to them, plants maintain their mystery. I might not handle them daily anymore, but I like to think of them growing in the fields and waysides of the world: rue and cockspur, nettle and rosemary, rising from the soil year after year to spell out a code we may not ever completely crack.
The problem of the bicameral mind
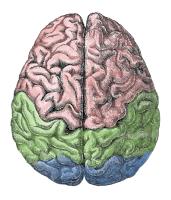
One is the qualitative, enumerative, reductionist particularistic view of causation: causes are individual forces or 'things' and the job of science is to identify them and how they work. To do that, we have to isolate them, and to do that we must reduce our observations to the most rudimentary level--such as molecules, where the causes actually operate. We do this through experimental designs and so on. We do it because complexity obscures individual causes. This is the gene mapping approach, the number-crunching idea that we'll just overwhelm Nature with our computers and force it to yield its individual causes. Mendelian genetics has been an exemplar of this worldview since the turn of the 20th century. It assumes great regularity in Nature, and that the scale of our data and so on are all that prevents us from identifying causes.
The other view is quantitative, and basically holds that Nature works through complex interactions at higher levels than rudimentary forces. A building cannot be understood by enumerating its bricks, beams, and wires, even if those things are needed and in that rudimentary sense 'cause' or explain the building. But interactions of very complex forms are instead viewed by quantitative minds as the organizing principles, or higher-level 'causes', that we need to understand. Quantitative genetics as separate from Mendelian genetics has always been a major component of biology, and was basically Darwin's view of evolution. It treats genetic and evolutionary causation in aggregate, without attempting to enumerate its components. This view today, though currently held by the vast minority because it's under siege by the reductionist majority, would be that which argues that computer crunching is not what we need: what we need is more innovative thinking about how complex causation works and/or is to be understood and manipulated.
This qualitative/quantitative dichotomization is an oversimplified characterization, and we don't mean to suggest that the world is just a contest between opposites that will ultimate resolve (that's a view of philosophers such as Hagel, and thinkers like Marx). Still, it reflects widespread differences in world views--of communication between our two brain hemispheres, one might say.
There are attractions and attributes to these different points of view and their intermediaries. How they will resolve, or if they will, remains to be seen.
No comments:
Post a Comment