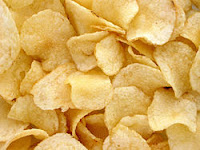
The researchers did a meta-analysis of 100 studies of the risk of mortality associated with BMI, or body mass index, an indicator of how much fat a person is carrying independent of his or her height. A 'meta-analysis' pools results from many different studies, each of which may be too small in size to be definitive, in order to have larger samples and gain greater statistical power. In this case, the total included about 3 million people. Of course, one must assume that the different study samples are similar enough in their risk characteristics for this kind of pooling to be legitimate.
Be that as it may, the authors in this case found that subjects with intermediate BMI's, who are considered overweight but not grossly obese, with a BMI between 30 and 35, had lower risk of all-cause mortality than those with "normal" BMI of 25-30, or grades 2 and 3 obesity, BMI over 35. According to the JAMA press release about the study:
The researchers found that the summary HRs indicated a 6 percent lower risk of death for overweight; a 18 percent higher risk of death for obesity (all grades); a 5 percent lower risk of death for grade 1 obesity; and a 29 percent increased risk of death for grades 2 and 3 obesity.Why? Well, the researchers have numerous possible explanations, again as quoted in the press release:
“Possible explanations have included earlier presentation of heavier patients, greater likelihood of receiving optimal medical treatment, cardioprotective metabolic effects of increased body fat, and benefits of higher metabolic reserves.”That is, in part, BMI is likely to be a confounder, standing in for ('correlated with') other unmeasured variables that are the actual reasons that mortality is lower among overweight and moderately obese people, such as that they go to the doctor more and receive more treatment than thinner people.
And, the story in the NYT quotes physicians saying that it's not BMI that counts anyway, but other health indicators such as cholesterol levels, blood pressure or blood glucose levels. If those are normal, a person doesn't need to worry about losing weight for the sake of their health -- but, they are more likely to be elevated in people who are overweight. Illness can cause weight loss, too, so the population of people with "normal" BMI may well include some who are seriously ill. And, the location of the fat, whether in the belly or superficial, seems to be important too, and it varies from person to person.
The researchers do suggest that body fat might have protective benefits as well, and that may be so. But, the upshot of this study seems to us to be that the association of body weight with mortality risk is not straightforward. It's possible that in some people body fat is even protective, and more is better -- up to a point. And, well, if you know all sorts of other things, including whether the person already has symptoms or problems, then BMI becomes a useful predictive measure. In other words....despite expensive and extensive decades of research and countless news stories, and countless counselors, fad diets, exercise and reducing programs, and all the magazine and infomercial pressures on body weight, well, we're not so far from square one. Except, is it reasonable to say, for a lot of researchers who have made a lot of hay out of this for decades?
In the end, the decision as to whether or not you keep your resolution to lose weight should not be decided on the basis of this one study. We'll have more to say about this tomorrow.
13 comments:
And then BMI is often not a reliable indicator of fat because a very strong person's BMI could be elevated because muscle weighs more than fat.
You raise but one of many points, that are easy for a research establishment in a hurry to overlook or minimize or 'regress' out statistically.
To really estimate fat one needs more complex and costly and less easily compared measures, or even x-ray imaging.
And is it the fat that's important? Maybe muscle is an issue?
We'll have more to say about this in a forthcoming post.
The authors of the paper, as quoted in the NYT and elsewhere, do seem to recognize that it's not at all clear what their results mean, if anything. Which leads me to wonder why even write the paper? And certainly, why allow all the hype?
my strong-as-an-ox husband freaked out for months (years?) after a routine checkup when they told his his BMI was a bit high. Our PT friend tried to explain it all to him, but he believed the number, no reason. Ridiculous, that the doctor's office would allow someone who is all muscle to listen to that, but I imagine he didn't question it when he was there.
It's one of many ways in which glib story lines can lead to dangerous or disturbing misperceptions. Much better for people to acknowledge fully when something is simply not understood.
Clearly that's the case today, even after gobs of research, when it comes to obesity and related traits. Something's obviously relevant, but just as clearly we don't really understand what that is.
The same is true for many other common diseases, unfortunately, but the same 'omics' approach, easy to do and get funding for, distracts us from the serious and sobering challenge of finding better ways to study these traits, or, perhaps, to define them in the first place so they can better be studied.
It's kind of funny that, even though the topic is weight, investigation into nutrition doesn't seem to be heavily considered. Are there certain styles of diet that tend to promote obesity, diets which not only provide too much of a given substances (e.g., carbs) but too little of other nutritive factors? Are people who are only a bit overweight more likely to be eating a more balanced diet? Are underweight people prone to starving themselves of proper nutritional intake?
Those were some of the questions that struck me. Our society is very carb addicted, and then we also-- when dieting-- tend to avoid fats. While avoiding trans fats sounds like a good idea, avoiding fat content in general sounds potentially hazardous being that it is a necessary building block of the cell.
There are as many sure-thing answers to these questions as there are investigators and pundits to write about them. Every element of diet, maybe even water, has been blamed. Take your pick.
Anne keeps closer watch on this than I do, and hopefully she'll respond.
The key question, to me, is how on earth we could possibly not have clearer answers. And to me the 'answer' (or, better, explanation) has to do with our very inadequate ability to deal with complexity by taking reductionistic approaches to it. That sort of reductionism is in a way so deeply embedded in our scientific thinking that we have a hard time seeing differently. Maybe we should....but how?
Yes, those are all good questions, insolemexumbra, but unfortunately, to my mind, essentially unanswerable. The reasons are well-known and generalizable to epidemiology, genetics, and so on but generally ignored, no doubt because what to do instead is hard to envision. And, nutrition is particularly difficult to study because diet is so hard to assess; people have a difficult time estimating how much they eat of various foods, or they lie, or questionnaires are based on recall, which is notoriously unreliable. And so forth.
But the larger issues are that, 1, population studies yield average answers, and everyone is unique, not average. So, how to apply results to individuals, including risk prediction, is pretty near impossible to say. 2, risk factors, including genes, generally have small effects, and so are hard to detect. Smoking is a rare exception, and even its effects aren't uniform or entirely predictable. 3, everyone gets to their own phenotype, including weight, in their own way; different diet, different activity levels, on a unique genetic background.
As Ken says, we're trying to reduce risk to single factors, but it's worse than that; we're also trying to apply risk, generally of single variables, assessed at a group level to individuals. And, this most often requires ignoring how risk factors interact. So, we've got piles of group data on risk factors generally with small effects, all of which, by definition, ignore confounding -- unmeasured -- variables, and about which we're pretty much clueless as to how to make sense of it all on the individual level.
Is there a solution? Personalized medicine or genomics won't be it, as risk estimates are still based on group data, with all the above problems. And simplistic studies, such as the BMI/mortality study we write about here, are not it either.
And I'll just add a caveat emptor to Anne's last comment. Beware the post-hoc claims made to justify work. There will always be some genetic (or environmental) risk factors that do have some predictive power. These 'successes' are routinely touted rather loudly to justify continuation of business as usual, even if most attempts failed. It avoids the questions whether there could have been more effective ways to expend funds and effort, and whether there are entirely different and better ways to conceive the problem in the first place.
"As Ken says, we're trying to reduce risk to single factors, but it's worse than that; we're also trying to apply risk, generally of single variables, assessed at a group level to individuals. And, this most often requires ignoring how risk factors interact. So, we've got piles of group data on risk factors generally with small effects, all of which, by definition, ignore confounding -- unmeasured -- variables, and about which we're pretty much clueless as to how to make sense of it all on the individual level. "
Would a good summary of these problems of confounding factors and and unique genetic backgrounds be: additional uncertainty that arises from applying average population-level answers to individuals? If so, this makes me wonder if hierarchical statistical modelling could help here? More generally, I think we might need more 'honest' statistical methods? What I have in mind are methods that report population-level findings (and associated uncertainty) along with some measure of the additional uncertainty that arises when someone wants to understand the relevance of these findings for themselves. Effectively this would essentially mean widening the error bars. Studies should say something like 'for the average person we understand the effect of factor X on disease Y with level of confidence Z, but for any particular person this level of confidence should be reduced by amount W'. I think the fairly well-established field of hierarchical modelling could help here. Indeed this field is essentially statistical sciences answer to all extrapolation problems (e.g. http://andrewgelman.com/2012/06/hierarchical-modeling-as-a-framework-for-extrapolation/)
Of course the problems are: (1) its much easier to sell your message when you forget about W and the caveat about the 'average person', and (2) communicating the results of hierarchical models to a general audience is difficult.
So, currently we've got things like equations that allow us to estimate our risk of having a heart attack based on known risk factors, such as whether we smoke or are overweight. That is, applying population level data to individuals. (Though, I've never understood what, say,"30% risk" actually means.) But, are you suggesting that if we widen the error bars to -- correctly -- acknowledge that because of unmeasured variables, including unique genetic backgrounds, such a risk estimate might be more meaningful?
I'm not statistician enough to be clear about your suggestion, though they are clearly constructive and well thought out. I don't know the basis of the hierarchical modeling you are referring to, but if it means identifying the strongest factor first, then evaluating risk in those individuals with that factor in terms of their possession of lesser factors, I think this is going to be a multivariate mess for many reasons.
Jason Moore at Dartmouth has been toying with various models that may be relevant and you might write to him.
I think this is different from confounding due to unmeasured (or unknown) causal factors. The latter introduces additional variance of the sort I think you're referring to, if the causal and confounding factor are only weakly correlated.
But I'm not clear if you've distinguished confounding with interaction among measured factors. Also, how many levels of interaction can one accommodate and how does one begin to assess this. You might look at Eric Lander and others (I forget the other authors' names, but the lead is Chinese as I recall) advancing the idea that it is interaction that accounts for 'hidden' heritability. But even there it is obvious that making sense of interactions is going to be a challenge.
Many if not most models assume homoscedasticity which I think can fairly be characterized is that for each risk factor, variance in risk is the same. Thus the distribution among individuals is estimable in a crude way, but his/her risk is only a point (average) estimate plus some variance--I think you are referring to this in a way as well. But what do we assume about that variation?
In a paper long ago, Anne and I noted that a group risk of n% doesn't at all mean all in the group have that same risk. A major player in this game, David Altshuler, has also discussed the issue of how to evaluate the variation in risk of members of a group--such as bearers of some specific genotype.
How one wades through all of this even with hierarchical statistical models is beyond my abilities to know (or judge). But if each step increases variance on risk estimates, and if the net result is that the point (average) risk is essentially useless---then why are we still approaching things this way??
Finally, I would note something I say in my talks all over the place, but nobody ever really wants to think about, which is that when environments are part of risk (as they always are), then risk estimates, which are inherently retrofitted to past exposures, are useless if we don't know what future exposures will be....and we never do!
So to me, statistical and mathematical models are designed historically for replicable phenomena, with proper mathematical or probabilistic properties (like rolling dice), but this simply is inapt with regard to much that makes up the areas of epidemiology that are involved here.
Also, this may be an instance of the well-known 'ecological fallacy' of assuming that risks estimated for groups apply to individuals in the groups.
In genetics, programs exist to try to remove internal structure in groups as false sources of this sort of error. A recent paper by authors including Magnus Nordborg -- you can easily find it, I think -- concerns some of these issues, of how important group substructural heterogeneity is responsible, or not, for GWAS kinds of association studies. You might benefit from reading their arguments.
To me, perhaps reflexively skeptical at this point, I think that trying to find a formal statistical way out of an epistemological box may be inevitably frustrated as being rather inappropriate. And the ontology (actual reality) may require a different epistemology (inferential approach).
But these, of course, are rather useless kinds of statements!
Post a Comment