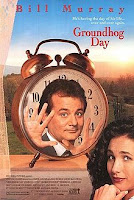
Is it just being curmudgeonly to ask these questions? Or is it that where some see irreducible complexity others see a simple explanation that actually works?
An isomorphic problem
The important thing about these various issues in modern science is that from the point of view of gaining knowledge about the causal world, they are isomorphic problems. They have similar characteristics and are (currently) addressed by approaches with similar logic--in terms of study design, and similar assumptions on which both study design, data collection, and methods of analysis are based. The similarities in underlying causal structure include the following:
Often, people use the word, but persist in applying enumerative, reductionist approaches that we inherited over the past 400 years largely from the physical sciences (we've posted on this subject before). This is based essentially on the repeatability of experiments or situations. We try to identify individual causal elements and study them on their own. But if the nature of causation is the integrated effects of uniquely varying individuals, then only the individual strong (often rare) factors will be easily identified and characterized in this way.
Item #8 above is important. In physics we have strongly formal theory which yields precise predictions under given conditions. There is measurement error, and the predictions are sometimes probabilistic, but the probabilities involved and the statistics of analyzing error, were designed for such situations. We compare actual data to predictions from that externally derived theory. That is, we have a theory not derived from the data itself. It is critical to science that the theory is largely derived not just in our heads but from prior data. But it's external to new data that we use to test the theory's accuracy.
In the situations we are facing in genetics, evolution, biomedicine, and health, we have little similar theory, and the predictions of what we have are not precise or our assumptions too general. Even the statistical aspects of measurement error or probabilistic causation are not based on rigorously specified expectations from theory. Our theory is simply too vague at this stage. So what do we do?
We use internal test criteria. That is, we test the data against itself. We compare cases and controls, or different species of apes' skeletons, or different diets. We don't use some serious-level theory to predict that so many eggs per day, or some specific genotype at many sites in the genome will have some specific effect based on primary biological theory, but only that there is a per-egg outcome. We don't know why, so we can't really test the idea that eggs really are causal, because we know there are many variables we just aren't adequately measuring or understanding. When we do find strong causal effects, however, which does happen and is our goal of this kind of research, then subsequently we can perhaps develop a real theoretical base for our ideas. But the track record of this approach is mixed.
This is also often called a hypothesis-free approach. For most of the glory period in science, the scientific method was specifically designed to force you to declare your idea in a controlled way, and test it (the 'scientific method'). But when this didn't work very well, as in the above areas, we adopted a hypothesis-free approach that allowed internal controls and tests: our 'hypothesis' is just that eggs do something: we don't have to specify how or why. In that sense, we are simply ignoring the rules of historically real science, and even boasting that we are doing science anyway, by just collecting as much data as we can, as comprehensively as we can, in the hopes that some truth will fall out.
The central tenet of science for the last 400 years has been the idea that a given cause will always produce the same effect. Even if the world is not deterministic, and the result will not be the same exact one, it will at least have some probability distribution specifying the relative frequency with which we'll observe a given outcome (like Heads vs Tails in coin-flipping). But we really don't even have such criteria in the problems we're writing about. Even when we try to replicate, we often don't get the same answer, and do not have good explanations for that.
An isomorphic problem
The important thing about these various issues in modern science is that from the point of view of gaining knowledge about the causal world, they are isomorphic problems. They have similar characteristics and are (currently) addressed by approaches with similar logic--in terms of study design, and similar assumptions on which both study design, data collection, and methods of analysis are based. The similarities in underlying causal structure include the following:
- Many different factors contribute causally to the outcome
- Most of the individual factors contribute only a small amount
- The effect of a given factor depends in various ways on the other factors in the individual
- The frequency of exposure to the factors varies greatly among individuals
- Sampling conditions (how we get the data we use to identify causal elements) vary or can't really be standardized
- The conditions change all the time
- The evidence for causation is often indirect (esp. in reconstructing evolution)
- We have no underlying theory that is adequate to the task, and so we use 'internal' criteria
Often, people use the word, but persist in applying enumerative, reductionist approaches that we inherited over the past 400 years largely from the physical sciences (we've posted on this subject before). This is based essentially on the repeatability of experiments or situations. We try to identify individual causal elements and study them on their own. But if the nature of causation is the integrated effects of uniquely varying individuals, then only the individual strong (often rare) factors will be easily identified and characterized in this way.
Item #8 above is important. In physics we have strongly formal theory which yields precise predictions under given conditions. There is measurement error, and the predictions are sometimes probabilistic, but the probabilities involved and the statistics of analyzing error, were designed for such situations. We compare actual data to predictions from that externally derived theory. That is, we have a theory not derived from the data itself. It is critical to science that the theory is largely derived not just in our heads but from prior data. But it's external to new data that we use to test the theory's accuracy.
In the situations we are facing in genetics, evolution, biomedicine, and health, we have little similar theory, and the predictions of what we have are not precise or our assumptions too general. Even the statistical aspects of measurement error or probabilistic causation are not based on rigorously specified expectations from theory. Our theory is simply too vague at this stage. So what do we do?
We use internal test criteria. That is, we test the data against itself. We compare cases and controls, or different species of apes' skeletons, or different diets. We don't use some serious-level theory to predict that so many eggs per day, or some specific genotype at many sites in the genome will have some specific effect based on primary biological theory, but only that there is a per-egg outcome. We don't know why, so we can't really test the idea that eggs really are causal, because we know there are many variables we just aren't adequately measuring or understanding. When we do find strong causal effects, however, which does happen and is our goal of this kind of research, then subsequently we can perhaps develop a real theoretical base for our ideas. But the track record of this approach is mixed.
This is also often called a hypothesis-free approach. For most of the glory period in science, the scientific method was specifically designed to force you to declare your idea in a controlled way, and test it (the 'scientific method'). But when this didn't work very well, as in the above areas, we adopted a hypothesis-free approach that allowed internal controls and tests: our 'hypothesis' is just that eggs do something: we don't have to specify how or why. In that sense, we are simply ignoring the rules of historically real science, and even boasting that we are doing science anyway, by just collecting as much data as we can, as comprehensively as we can, in the hopes that some truth will fall out.
The central tenet of science for the last 400 years has been the idea that a given cause will always produce the same effect. Even if the world is not deterministic, and the result will not be the same exact one, it will at least have some probability distribution specifying the relative frequency with which we'll observe a given outcome (like Heads vs Tails in coin-flipping). But we really don't even have such criteria in the problems we're writing about. Even when we try to replicate, we often don't get the same answer, and do not have good explanations for that.
When we're in this situation, of course we can expect to get the morass of internally inconsistent results that we see in these areas, and it's for the same basic epistemological reason! That is, the same reason relative to the logic of our study designs and testing in these very different circumstances (genetics, epidemiology, etc.). Yet that doesn't seem to slow down the machine that cranks out cranky results: our system is not designed to let us slow down to do that. We have to keep the funds coming in and the papers coming out.
And then of course there's cause #9. Most of us have some underlying ideology that shapes our interpretation of results.
This is all a fault of us and the system. We can't be faulted for Nature's complexity. The issues are much more--yes--complex than we've described here, but we think this captures the gist of the problem. Scientific methods are very good when we have a good theory, or when we are dealing with collections of identical objects (like oxygen or water molecules, etc.), but not when the objects and their behavior are not identical and we can't specify how they aren't. We all clearly see the problem. But we haven't yet developed an adequate way to deal with it.
And then of course there's cause #9. Most of us have some underlying ideology that shapes our interpretation of results.
This is all a fault of us and the system. We can't be faulted for Nature's complexity. The issues are much more--yes--complex than we've described here, but we think this captures the gist of the problem. Scientific methods are very good when we have a good theory, or when we are dealing with collections of identical objects (like oxygen or water molecules, etc.), but not when the objects and their behavior are not identical and we can't specify how they aren't. We all clearly see the problem. But we haven't yet developed an adequate way to deal with it.
4 comments:
I think many of these comments apply to ecology problems as well.
These comments most likely apply to any field trying to explain complex traits/events. That is, just about any field. Economists have recently discovered complexity, for example -- well, except for the ones who are trying to find genes for economic behaviors. Political scientists, except for those looking for genes for how you vote. So, yes, we apply them here to the fields we know best but that doesn't mean then are restricted to these fields.
I heartily agree with this stance, but ... in the interests of challenging ourselves, I think it's worth re-reading Platt's 1964 "Strong inference" paper and asking ourselves whether we are sometimes sucked into embracing complexity prematurely, rather than trying to think hard about the logical structure of what we're doing. (The wikipedia article contrasts the strong-inference/alternative-hypothesis approach with the single (vs. null) hypothesis approach, interesting to think about how alternative hypotheses contrast with *no* hypotheses ...)
(having trouble posting the wikipedia link, but it should be easy to find)
doi:10.1126/science.146.3642.347
It is a good theory that reduces complexity to something simpler or at least more systematic. I think that we either don't yet have such a thing for many current problems or phenomena, or our idea of what a 'theory' is needs to be reformed.
Some areas may not be apt for universal theories of any simple 'formulaic' theory.
Post a Comment