The terms are defined in circular ways (random means haphazard or due to chance) or in terms of events that were repeated, or that might be repeated in the future, or the fraction of those events with some particular property, or even of events that may not in fact occur or even be possible. Or in terms of what might 'possibly' (another vague term) happen in the future. Or how convinced we may be that it will happen.
Probability and statistics are at the very foundation of modern science. But as specialists know and confess readily, the central terms are vague and in a formal sense, axiomatic. You define a probability as a number between 0 and 1 that represents something related to the concepts mentioned in the previous paragraph. An axiom is accepted and used, but not directly tested and need even not be a part of the real world. 2+2=4 is loaded with such kinds of assumptions.
Probability seems so deeply embedded, and just plain obvious, that it's hard to accept that its use and real-worldliness can be boiled down to beliefs or to something that we just take for granted rather than test. Even something as simple as rolling dice shows the issues, and they're important because they are seen all over the place in human and evolutionary genetics.
From: Ivar Peterson's Math Trek
Let's look at some tests done with dice. Here are results from a web site tallying the rolling of 10,000 dice. Now, the natural reaction is to assume that the spots on each face make no difference to whether it will end on top on a given roll. Somehow we naturally then assume that in 10,000 roles we expect 1667 occurrences of each face. This was not always an obvious expectation, but it has been since WRF Weldon rolled 26,306 dice in 1894, which led to the still-current way we interpret such results.
Let's look at some tests done with dice. Here are results from a web site tallying the rolling of 10,000 dice. Now, the natural reaction is to assume that the spots on each face make no difference to whether it will end on top on a given roll. Somehow we naturally then assume that in 10,000 roles we expect 1667 occurrences of each face. This was not always an obvious expectation, but it has been since WRF Weldon rolled 26,306 dice in 1894, which led to the still-current way we interpret such results.
Clearly, the expected result is not what happened! Does this--the actual results, not anything 'theoretical'--then mean that the dice are biased in some way? Nowadays we would all be unclear until we do some kind of statistical test. Following what Karl Pearson developed from Weldon's experiments, we compare the above results with 1667 for each face and say yes, the two are different, but ask how different and whether it matters. We use some subjective goodness-of-fit test cutoff level to evaluate the difference, such as are routine in science and taught in basic statistics courses.
If the subjective cutoff is exceeded, then we say that if our idea that, for whatever reason, each face should come up an equal number of times, the results are unusual enough that we doubt our idea. A typical cutoff would be that if the difference would be as great as what we see in less than one experiment out of 20 experiments, we say our idea is not acceptable. Note that this is purportedly a scientific approach, and science is supposed to be objective, but this is a wholly subjective choice of cutoff, and it assumes a lot of other things about the data (such as there was no cheating, each toss was done the same way, and so on). Weldon's dice also seemed unfair, but in unclear ways, if one thinks of the possible reasons for unfairness. They even wondered if one of the assistants doing the rolling might have done it differently.
This seems strange. We might decide that the dice are unfair in this subjective way, though that doesn't tell us how or why they're unfair. But in another sense, the differences are numerically so small that we might say 'who cares?' (Las Vegas gambling houses care!)
But notice something: on dice, the spots on opposite sides total to 7. Thus one side has more spots than the opposing one. For example, 1679 sixes vs 'only' 1654 ones. This is true for all such pairs, even if the individual differences don't seem startlingly great. But the above data suggest that since the spots are really dips in the surface of normal dice, they take some mass away so that the weight of the dice is shifted from dead center towards the heavier (fewer spot) side. The more spots the lighter and the more often it comes up. Bingo! A physical explanation for an otherwise curious result! (I understand that spots on Vegas dice are filled with black material of the same type as the rest of the die).
The significance test that led us to this decision does not imply that the next 10,000 throws of these same dice would come up the same, but the usual thing in science would be (1) stick to the fairness belief and ignore the result, assuming that the next result would be 'better', or (2) adjust the expectations from 1/6th for each side to these observed fractions, and then test the next experiment against these expectations.
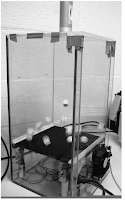
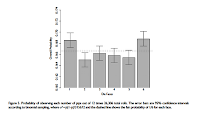
Note here that there are ways to establish ranges around our observed results that represent what might have occurred had the same dice been rolled the same number of times again (the brackets in the figure, for example, show this). But one has to choose the limits subjectively. The brackets would not be identical from experiment to experiment.
Even if you said that the results are not very different from each other, do you mean that they are not very different from 1/6 for all faces, or from the biased probabilities of the 10,000-roll experiment? Or from some other type of bias? Should you have a different amount of bias favoring the 6 from that of the 5 and the 4 (the lighter of their respective face-pairs)?
If this were something whose outcome affected you personally, you likely would say it doesn't matter, if you were playing Monopoly or shooting dice with friends. But if you're the MGM Palace in Las Vegas, you would care much more, because there what counts, so to speak, is not the money made or lost by individuals but by your entire customer base. That can be a very big difference!
One last thought here. The idea that we should expect each face to come up 1/6 of the time rests on the concept of 'randomness'. But that is an idea so elusive one can ask what it actually means. Normally the idea is that each die face is the same, and so there is 'no reason' any one should come up more often than another. That is essentially unprovable and very likely untrue. But at least, especially in Vegas dice with filled-in pips, the faces of a die are (if fairly manufactured etc. etc.!!) so similar that our intuitive concept is probably not so bad. We were going to say that, after all, most such things do come up more or less as expected....but we hesitated, because we'd have expected that with dice, too!
The same problems and infuriating (or intriguing) issues arise in something so simple as coin-tossing and asking whether a coin is biased. Normally we would consider it, like dice, to be a 'random' phenomenon and that's why heads and tails come up the same fraction of the time (if they do!). This raises other fundamental questions, as we'll see in Part III. There, we'll show how very relevant all of this is to human medical genetics, studies like GWAS, and to the inferences we make about evolution.
2 comments:
math trek link is broken.
his results look extremely suspicious to me: 4 or 5 throw differences between each of the numbers, in perfect ranking order? out of 10k throws if its a real effect it is very small and the noise inherent in the experiment makes me believe his perfect-pattern results are >99% fabricated ;)
perhaps mention mendel's suspiciously good results?
It really doesn't matter for the point. Weldon's original data, and the machine-tossed data all have their quirks and are ambiguous relative to some specific expectations. The center of mass theory was suggested, as I recall, by the flipper. I think I read somewhere in this context that this (pit-weight) is why Vegas dice are filled flat (I was at Vegas once, but didn't check out their dice)
Post a Comment